AI from Board Perspective
In this episode of the Fit4Privacy Podcast, we explore the evolving landscape of AI governance through the lens of boardroom discussions. Our guest, Chris Burt, a seasoned advisor to boards and expert in AI, offers valuable insights into the key considerations and strategies for navigating this complex terrain.
Transcript of the Conversation
Punit 00:00
AI from board perspective, yes, we've been talking about artificial intelligence or AI, sometimes the EU AI act in last few episodes. But what does the board so the board members or the executive leadership think about artificial intelligence? What are their interests? What do they care for? And how are they looking at artificial intelligence in terms of leveraging within the company and outside the company? And while we have that talk, I think it's important that we understand, and I think we all do that AI is relatively a new topic. So hence everyone is learning, and as everyone is learning, everyone is also discovering while they have the fear of missing out. So, while we all learn, let's learn from someone who is advising boards in many parts of the world, starting from the UK, and my dear friend Chris Burt, who's the principal and lead consultant lead in advising boards, in Helix Consulting. He's also in the risk coalition, and let's go and talk to him. What are the boards thinking about artificial intelligence? What are the concerns they have? Do they see privacy as a challenge and everything else around AI for board.
FIT4Privacy 01:26
Hello and welcome to the Fit4Privacy Podcast with Punit Bhatia. This is the podcast for those who care about their privacy. Here your host, Punit Bhatia, has conversations with industry leaders about their perspectives, ideas and opinions relating to privacy, data protection and related matters. Be aware that the views and opinions expressed in this podcast are not legal advice. Let us get started.
Punit 01:55
So here we are with Chris Burt, Chris, welcome to Fit4Privacy Podcast.
Chris 02:00
Thank you. Great to join you.
Punit 02:01
It's a pleasure to have you. Of course, we've been having quite a few conversations in last few months, and I'm really impressed with the way you articulate things toward the board. And if I may ask, in context of artificial intelligence or data privacy or the emerging technology, all these things that we talk about. How would you define maybe, let's say take for example, AI, artificial intelligence from boards perspective. Because for us, it's scary for us, for some both, and for some of us, it's money making. But how does the board look at AI?
Chris 02:41
Right? So, my experience with boards, so I work with several boards, and what I'm hearing, more than anything else, is FOMO fear of missing out. So, boards are very much aware of AI. They've discussed AI, and they're very keen to do something around AI. Question is, what? And that's the bit they struggle with. It's understanding, well, where can we use AI to our advantage without exposing the organization to significant risks? And there's this real fear of missing out. We need to do something. We're quite desperate to do. So don't use the words desperate, you know, essentially, they are desperate to do something and to be seen to be doing something as well. Because there's a market expectation that organizations will be embracing AI, because of everything we hear in the press, in the press about the importance of ai, ai and how it's going to transform the world. The boards and the organizations they represent want to be part of that, but they are nervous about exactly where they invest their time and their money and their effort and what the results might be. And of course, there are also the scare stories around data being inappropriately used or inappropriately secured or shared with people it shouldn't be shared with, and all those kinds of so there is a nervousness around do we actually understand this thing? And when speaking to boards, when I'm doing board evaluation work with boards, one of the things they quite regularly bring up is, we need to have some technology expertise on the board. We need some AI expertise on the board, which is a natural reaction, I think, to the challenge of technology and cyber and AI and emerging tech. But I don't think it's necessarily the right answer to that, but is, you know, is fairly natural response to those kinds of questions. So that's effectively what I'm saying at the moment.
Punit 04:50
No, so fair enough. There is a fear of missing out that everyone has, and it's natural to have it. There is this desire or intention to do something about it and leverage. For the company, that's also okay, and there's also a bit of anxiety, because they don't probably understand what is AI for them. But initially, in the early conversations, what kind of understanding of Ai do they bring? Do they see it as a risk, as an opportunity? Or they are neutral early on.
Chris 00:00
Both they they've seen the press. They've seen ChatGPT turned everyone's world uptime upside down and changed everyone's perception of AI. So AI has been bubbling away in the background for a long time, and people have been vaguely aware of it, but ChatGPT came along and changed everyone's perception of AI and what it's capable of, and it's really opened eyes. And so there is a real appetite to do things with the organization, to change the way that it works. And they're hearing knowledgeable people talking about this is the next revolution. We've had, the Stone Age, the Iron Age and blah blah industrial revolution, all the rest of it, and AI and all the supporting technology around that their hearing is going to be the next revolution. But again, we come back to what is it that we do? So there's certainly bits of the organization where use of AI is obvious, where you can so, so, for example, we're hearing stories of Company Secretaries. For example, company secretaries, they sit in board meetings, they take notes of board meetings. And we're hearing stories of company secretarial teams wanting to use AI to produce board minutes. You know, fairly small but important activity. It's not going to transform the organization. But just using that as an example, we then explore, well, how good is AI at producing board minutes? And the answer is pretty good, 80% okay, but there are some challenges around it. Firstly, you have to give the AI tool access to the board meeting. So that's either a recording or real time access to the meeting. What happens to the recording of the meeting? Does that become a company record, and can you destroy it, or is it not a company record? And the minutes that are actually finally produced are those the company record? So there's some lack of clarity around how you actually produce the minutes. And then there's the question around what is actually produced. So AI will produce reasonably good quality board minutes, as someone described him recently, AI gives you a solid B minus in terms of the quality of something. Is not a straight A is A B minus. So there's always something not quite right, and what AI isn't good at, which is what an expert is good at, is nuance and having an awareness of the surrounding context. So an experienced company secretary can be sat in a board meeting, and we'll realize that actually this conversation is really rather sensitive, and I can't go into too much detail, so I'll lift the minutes of this conversation up a level, no, so that it's still technically minutes, but not into the detail of, Hey, I can't do that. And so and so. What we see is actually AI is a useful tool to support experts and to accelerate work by doing a lot of heavy lifting on behalf of an expert, but you still require the expert to produce the final product, leveraging what the AI has done. That's a simple example, but that's what organizations are doing. They're kind of looking around their organization, trying to figure out, what is it that we can do? And organizations are taking different approaches to figuring out what they can do. So one of the things that I speak to organizations about is to use a principles based approach, a tried and tested approach. So, I mean, I'm very old, so going back to the early 2000s we had Sarbanes Oxley come along from the United States, where you had to essentially document your processes, document your risks, document your controls, test them, and all this kind of stuff, very bureaucratic, very labor intensive. But one of the things that came out the back of Sarbanes Oxley was the issue of spreadsheets and end user computing, and the fact that we realized that we had to identify all of these spreadsheets that ended up going to the financial reporting process, and we had to identify all the end user computing which influenced the end the financial reporting process, and we had to wrap controls around all of this stuff so that we couldn't get spurious information going into the external financial reporting process. It all had to be controlled. And whilst that was painful at the time, once it was because. Controlled once we knew which were the critical spreadsheets, what the critical controls over change controls were needed over the spreadsheets, and where they should reside, and all that kind of we had that all played it's all fine work. Well, to my mind, we're kind of in the same position with AI. So the process that I encourage organizations to go through boards to go through is to number one, to address this, this FOMO thing, have a blue sky board discussion, or several blue sky board discussions on AI, what is it that we want to do broadly speaking? What is it that we want to avoid doing broadly speaking, and to have that, that conversation, that discussion, without the clock ticking, we've got half an hour on this paper, we need to make a decision, move on, because board meetings are very we need to make a decision, move on. We need to make a decision, move on. And they're always against the clock. So this discussion needs to be outside of a normal board meeting, so there is less time pressure, and the board members need to have time to come into a broad consensus amongst the group as to what we want to do and what we don't want to do, and some of the some stuff in the middle when we're not quite sure what we want to do in this little bit. And from there, you can then start saying, Well, okay, well, how much risk do we want to take in doing this stuff? Yeah, the stuff we want to do, how much risk we want to take in doing them and that should then provide the senior management team with sufficient information to be able to go away and document a a board policy on AI and emerging tech. So this is going to be our board policy on AI and emerging tech. Here is our AI strategy. Here's how much is our risk appetite, how much risk we're willing to take. Here's the stuff we are going to do, here's the stuff we're not going to do. Here are the material controls over those risks. So the so we understand what controls we need, and that policy is then central to everything the organization does around AI. So this is, again, we're going back to normal approaches in dealing with this stuff. This isn't all rocket science. This is a standard kind of approach. Come up with a policy, just come with a strategy, come up risk appetite, do that stuff. From there, you then start to implement the policy. And of course, one of the things that you need to do with AI in implementing AI is to understand, well, what ai do we have already, and we do we have copilot hanging around, but we didn't know that we approved all this kind of stuff. AI is popping up everywhere, and not necessarily in a controlled way. So guess what? We need to do. We need to understand what AI we have. Where is it? How is it being used? Who's controlling it? Where does the data go? How are we maintaining controls over that data? And good old fashioned spreadsheets, we can put in a spreadsheet, or however you want to do we need to have a register, a log of all this stuff. And from there we can then say, right, okay, so we've got, we've got all this stuff already in the organization. Some of this stuff is higher risk. Some of this stuff is lower risk. Of the higher risk stuff. What controls we have in place? We need to add more controls, or whatever else. We need to stop doing this. We need to whatever it is so that that enables the organization to at least get control of its current usage of AI, which, quite frankly, there will be end user computing style use of AI people were using ChatGPT to use to help them write stuff, and Heaven knows what else. And because there's no policy in place, there won't be any particular rules in place as to whether they should or shouldn't be using ChatGPT to help and write stuff, and there will be an element of experimentation amongst people within the organization, they're experimenting with using stuff. And is, am I implementing software? I don't think I'm implementing software. Therefore the controls around implementing software don't apply. I can just go and use this stuff, because it's a service that I'm just using web browsers. Web browser, and I'm using Twitter, and so a lot of it will be uncontrolled, and a bit, you know, we don't know how much risk we're facing because we don't know how it's being used. So part of the process is to understand how it's currently being used, who's using it, whether doing it, doing with it, all those kinds of things. And once you've kind of established control over what you're currently doing, the next thing to figure out is, well, okay, so we're now under control. We're not going to blow up, we're not going to lose our data, we're not going to end up on the front pages of the newspapers for all the wrong reasons. Now let's really start to explore how we can transform this organization, looking back at our AI strategy and our risk appetite and all those types of things, and so what I encourage organizations to do is to look at setting up. Some people call it a skunkworks or accelerator or an incubator or a center of excellence. Some. Place that is able to bring together people from across the organization to understand what they do, how they do it, and whether there's a potential to introduce AI into some of the things that they do, and what would be the benefits. So this is a typical new product development time process. And again, yeah, we have new product development processes. They've been established for many established for many years, and it's just applying that kind of mindset, that thinking, to AI, yep, so AI, yes, it's new, but we have all the ways and means of dealing with this stuff in a controlled manner, and that's what we encourage people to do. So it's that structured approach and go. And once you've kind of got your center of excellence up and running, they can then be producing regular Board Papers telling the board this is what we're doing. We've explored this. It didn't work. We've explored this. This has potential. In line with what you've said, we're not going anywhere near this stuff because he told us not to. And the board might want to revisit the organization's strategy. Might want to revisit the risk appetite, but it's doing it in a controlled manner. The board is aware. What's happening is, is providing governance and oversight and challenging man, changing management on what they're doing, how they're doing it, and what the results are, which is how a board should be working.
Punit 16:27
Yeah so, I think we are talking about in three broad things. One is getting the board to understand, or know, what is this AI, and what can they do? Because not everybody understands it. So, bringing them on the same page, then getting them to frame a policy, but policy in two dimensions. One being, how do you leverage AI to create efficiencies, gain time and maybe optimize the use of resources inside and the third is, or the second is, how do you use AI to create competitive advantage for you in terms of maybe introducing a product or introducing AI into your existing product life cycle, or into your existing product, and then develop that policy. But the question would be, it's all fair and it's all logical, but who does it? Would it be a consultant, like you and me, or would it be the internal team, or would it be a mix of internal us and someone else?
Chris 17:24
Well, the first answer is always me, but obviously most cases probably not. I think use of consultants is important, but they need to be the right consultants. There's I'm hearing crazy numbers from some of the consultancies in terms of percentage of revenue that they expect to generate through AI consultancy, and that's absolutely fine, but that's almost like having someone come into your organization and rip it to pieces. And I don't think that's the right approach. I think my personal view is that use of an experienced consultant to come in and to provide specific, focused advice and guidance in terms of the type of things that we've just been discussing. Yeah, that can be incredibly helpful. So not trying to take over the whole project, but giving them that high level expert, even kind of sitting with the board and facilitating some of those board discussions around this stuff based on experience and all those types of things. I do hear about organizations hiring chief AI officers. I think that's interesting. I don't know whether it's a machine or a person, but, you know, I use another example. A few years back, I started to see Chief Sustainability officers being appointed, and they've kind of fizzled out. And it does seem to me that organizations do seem to appoint chief such and such an officer to ball rolling, to bring resources together and to create critical mass and expertise and learning. And then once the thing starts with actually, it moves away from being a project or a program and into Bau. And once you get it into Bau, you don't really need a chief AI officer, and I expect that role to disappear. But I do think, you know, it may be sensible to have something like that. I mean, we've already mentioned the Center of Excellence, so you might want to have someone sat in the middle of the Center of Excellence, pulling all the strings, encouraging, whether, whether you put lots of people into a center of excellence and it then does everything, or whether it's more of a coordination and facilitation Center of Excellence where the wider organization is doing stuff in the smaller, lighter Center of Excellence is doing. I mean, that'll just vary depending on organizations and. Are the way they like to work, in the culture that they operate so but yeah, so I do think careful use of consultants can be helpful. I think indiscriminate use of consultants is going to be hugely expensive, and probably you won't get your money back on it.
Punit 20:18
That's true, and I think it's also about getting both to a level when they can act and when they have sufficient insights to act upon. And that's where, when you say the business as your usual, I think you do need, maybe not a head of AI, head of data and head of analytics. Maybe head of data analytics and AI can be better. And then you set up an incubation center which takes care of your innovation, sustainability, AI, and all those things together. And so that's the experimentation center, and that's more the organization Center, which makes sure the data is there, the right quality is there, and so on.
Chris 20:53
Absolutely and I think we need to think broadly about what organizations could and should be doing. If I step back, there are a number of organizations, a couple of the FTSE 100 these days. So there's in the UK, there's an organization called Experian, which is a credit reference agency. I think it's global now. It's a FTSE 100 that started out as a technology department of great universal, great universal stores, which was a great big retail organization, and they set up a little data center, and it outfit, which then grew and grew, and they floated it off and spun it off, and it became, you know, a footsie 100 in its own right. And we see this elsewhere with that type of thing. So I do think that was probably not a central objective of most organizations, the potential to start something and for it to take on a life of its own and grow and eventually to be separated and floated off. I think that is there, and we will see that over the coming 1020, years, we'll see not many, but a few of those things happening. I suspect, as people have gone into this, they've set up a center of excellence or an incubator, and they've put some money into it. Some have worked. Some of not the ones that have worked superbly. You then look at, well, okay, so this, this is providing us with service. It's actually, is there a business here where this thing could provide services to others? And suddenly it becomes, you know, it's own organization in its own right. So that, I think will happen. I don't think many organizations will start out with that objective. Right now, most organizations will be looking at it in terms of, what is AI? How can we use it within our organization? Which bits of our organization can we transform with this? And that's an important thing to think about, is, what is the objective? What are we trying to do here? Are we trying to take a head count if the if the aim is simply cost reduction. I think that's a bit short sighted. Let me be perfectly honest with you, if we look at AI, current state of the art, I said earlier, it gives you a solid B minus, yeah. So it can compete with the experts. So an expert can look at something produced by AI and say that's produced by AI because it's got these mistakes in it. But what AI can do is it can accelerate, and it can lift someone who doesn't know much about the topic to a solid B minus it can lift their understanding and their competence and help them do something more quickly and more effectively. So in terms of AI and how it can work. I think we're looking at those kinds of ways of working. You know, is, it is not going to put everyone out of work. In fact, it's probably going to push the requirement for expertise, because it doesn't produce a perfect answer. Therefore, you need the expertise to work with the AI outcomes output, to polish it and get it to the expert level. And it will lift people that aren't particularly great at this particular topic. And it will lift them. There will be a shakeout of employment, no doubt, but we don't yet know what that will look like. We don't think we've got any clear idea, but it's if you go and look back at the Industrial Revolution, which is probably the most recent, similar scale change. It changed countries hugely, but it moved people from agriculture and farming into cities and industrial production. It wasn't the end of the world. It was just a changed world, and I suspect that's what we're looking at again. So it will change the way the world looks and works, some ways good, some ways bad.
Punit 24:58
I think it's always the case that. There's always a balance. There's a positive and there's a negative. But when this aspect of AI, or leverage of AI is being talked about at board level, is there any concern or challenge or an understanding around the impact on privacy.
Chris 25:19
That's a real concern people at board level. I mentioned earlier that boards do recognize the lack of technology, cyber-AI, emerging tech expertise at board level, and they're all looking to bring that onto boards. And I think that's a valid concern and a sense or natural reaction. But board members, these are these are bright people. These are experienced people, and they do read around the topics. So, it's not that they don't understand AI at all. They do read up on this stuff, and they'll know enough to understand some of the weaknesses now, and they'll see the press headlines around stuff as well. So, there is a concern around data. You know, how will our data be used? How will it be kept secure? Where will it go? And you know, who has access to that and all those types of things. And there is also, when you get into a bit more of a technical discussion with boards that are able to have that technical discussion, you start asking questions around well, if, if your data is set within your organization, and you're only using all data from within your organization, that's fine, but I've never met an organization yet that was happy with this data. So, we know every organization has poor data quality. Some have some have less poor than others, but no one's ever happy with the quality of the data that they have, even deep pools of data. But typically, there are issues with it, and the AI will be using that data, and inevitably, if the quality of the AI outcome will depend largely on the quality of the data, or poor data, poor AI outcome, good quality data, better AI outcome, but not perfect and so you know, what data Are we using? And now if we're using public internet data, we have to be very well aware that that is full of holes, and so the quality of the AI answers may not be any good. And the other problem with AI is, obviously it takes data, it does something with it, it produces an answer, and then that gets squirted back into the data. Now, if you've got something which is producing a solid B minus not an A, it's squirting slightly poor quality data back in and it's kind of you've got this self-perpetuating problem of data quality. Now this, I'm sure, I'm sure people are working on this challenge and how to deal with it, but so this just demonstrates that data is a really, really important challenge around AI. What data are you going to use? Is it high quality? Will using AI with this data improve the quality of the data? Will it reduce the quality of the data? And if we want to use external data, how do we prevent our data going back out externally? And if it's if our stuff is going external, how do we know it's been appropriately protected? Now these are all data questions we've been wrestling with for many, many years. I mean, control and access to data is not a new problem. It's just being used, yeah, and it's just, it's just being used in slightly different ways. So, we, you know, we've, we've done a lot of work around this stuff in the past, but one of the, one of the difficulties, I'll give you an example of one of the difficulties I see. So, everyone wants access to lots of data so that AI can learn, but nobody wants to share their data, because this is our data. So I work as a consultant. You work as a consultant now, many, many years ago, doesn't didn't happen anymore, but many, many years ago, I would go and do a piece of consultancy work, and you produce, I don't know, a policy or something. Then at the end of the engagement, you would give everything to the client. You'd have a copy of the policy in your in your bag or your laptop, and you it's not for, you know, stealing it. You're just kind of borrowing it, kind of, so the next client that you go to who wants a policy, you can accelerate the piece of work. In fact, the next client benefits from the previous piece of work because you've got something that you can work with as a starter. And guess what? You typically improve that policy, because it's the second time you've looked at and you've improved it. And so consultants can act a bit like honeybees going for. Out of flower and pollinating and sharing ideas and actually improving the world for everyone. You just everyone's just got to be willing to let them do it. The problem we have at the moment is everyone's putting their arms around their data, and everyone's saying, no, you mustn't share data anymore. So as a consultant, I can no longer do that. Take the policy with me, which means the next client I go to that wants that policy, I'm having to write from scratch and whatever's left in my rather dodgy brain, so the second client's having to pay more for the same piece of work than they would have done previously, and no one's benefiting, and it's actually leading to a degradation of effectiveness and efficiency across the market, because consultants can no longer operate as they used to no so that's a question between private benefit and public good. Yeah, and I don't think people have quite wrestled with that sufficiently in terms of, it's actually in everyone's interest to share data as much as possible in terms of progressing AI. But if we don't, we're probably better off, personally, better off by not sharing it, but as a general population, we would be better off if more people would share data. But that's, you know, that's an existential public, government type of problem.
Punit 31:16
It's a paradox. We want the benefits, but we don't want to do the effort. And that's the same thing here in the data. But you don't want to share your data, and then you have privacy concerns. So how can it work both ways? And what I kind of synthesize from this conversation so far is there is a deep concern, deep desire and a deep fear of missing out, but in that it's not an easy job to figure out what to do and how to do it and how to govern it, and how to manage it and how to address these concerns. So it's not easy for boards as well, and they are also kind of figuring out, finding out what to do in this hype or the hysteria of AI and the role, but if you're helping them, and if somebody wants to talk to you about this help, because, as I see, we spent maybe 3035 minutes, and it's very difficult to navigate this topic From a hypothetical board perspective, but when in a specific industry, specific board, specific people, specific problem, then it's possible to provide some specific guidance, or reasonably specific guidance. And if somebody is looking for that, how can they reach out to you?
Chris 32:36
Well, that's easy enough. You can find me on LinkedIn, so it's Chris Burt on LinkedIn, or you can just Google my name I should pop up, or the company name, helix, helix consulting, H, A, L, E, X, we do board evaluation, work, governance, work, risk management, work, and if you can't remember any of that stuff, I'm also a co founder of something called the Risk Coalition, which produces leading practice guidance for boards and board risk committees. So if you can remember risk coalition, you'll see that I'm there somewhere, and you can contact me through that.
Punit 33:11
That's wonderful, and I think it is a relevant topic.
And people do need that guidance, that bit of nudge, bit of clarity and bit of information, sometimes it does create confusion, but in the end, confusion is the way to clarity in most cases, especially at board level, and that's how it starts. So, in view of the time that we have foreseen, I think I would say it's been a wonderful conversation. We delved into different dimensions, and if people want to learn more or ask more and maybe get some help, they can always reach out to you. Absolutely, yeah. And then from now, I would say, thank you so much for your help or input. It is wonderful to have you.
Chris 33:57
Thank you for inviting me.
Punit 33:59
It's a pleasure.
FIT4Privacy 34:01
Thanks for listening. If you liked the show, feel free to share it with a friend and write a review if you have already done so. Thank you so much. And if you did not like the show, don't bother and forget about it. Take care and stay safe. Fit for privacy helps you to create a culture of privacy and manage risks by creating, defining and implementing a privacy strategy that includes delivering scenario-based training for your staff. We also help those who are looking to get certified in CIPPE, CIPM and CIPT through on demand courses that help you prepare and practice for certification exam. Want to know more, visit www.fit4privacy.com that's www.FIT, the number 4 privacy.com. If you have questions or suggestions, drop an email at hello(@)fit4privacy.com, until next time.
Conclusion
The conversation with Chris Burt provides valuable insights into the evolving landscape of AI governance. As boards seek to harness the potential of AI while mitigating its risks, a structured approach, informed decision-making, and a focus on data privacy are essential. By understanding the challenges and opportunities presented by AI, boards can position their organizations for success in the digital age.
ABOUT THE GUEST
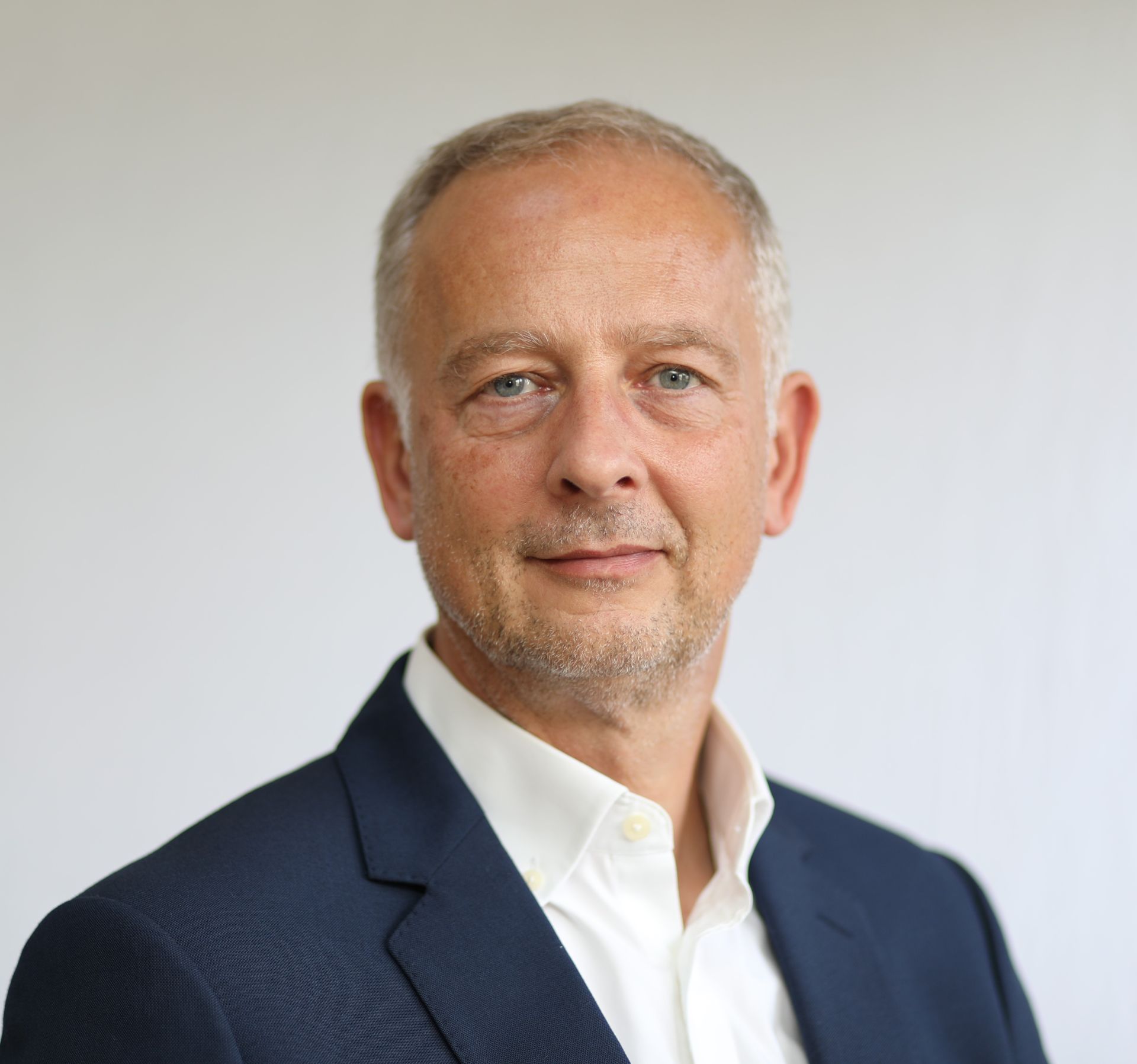
Chris is a CGI accredited board reviewer and Principal at Halex Consulting, a leading boutique governance consultancy specializing in independent board evaluations and risk management advisory services. He is also a co-founder and Exec Chair of the Risk Coalition, a not-for-profit, risk management think tank that aims to improve the effectiveness of risk management practice in the UK and globally.
Chris is the principal author of the Risk Coalition’s internationally acclaimed, ‘Raising the Bar’ leading practice guidance for board risk committees, and its soon-to-be-published ‘Raising your Game’ cross-sector leading practice guidance for boards and committees. He also Chairs the Risk Coalition’s Risk Committee Chairs and CROs forums, and Halex Consulting’s own Board & Committee Chairs and CoSec forums.
Chris speaks regularly on risk and governance-related topics.
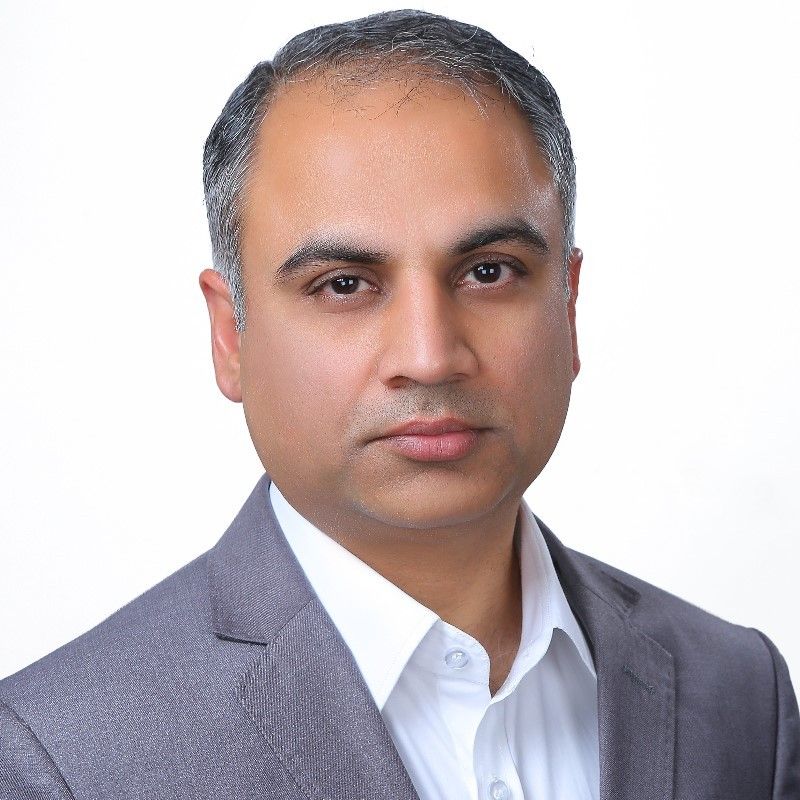
Punit Bhatia is one of the leading privacy experts who works independently and has worked with professionals in over 30 countries. Punit works with business and privacy leaders to create an organization culture with high AI & privacy awareness and compliance as a business priority by creating and implementing a AI & privacy strategy and policy.
Punit is the author of books “Be Ready for GDPR” which was rated as the best GDPR Book, “AI & Privacy – How to Find Balance”, “Intro To GDPR”, and “Be an Effective DPO”. Punit is a global speaker who has spoken at over 50 global events. Punit is the creator and host of the FIT4PRIVACY Podcast. This podcast has been featured amongst top GDPR and privacy podcasts.
As a person, Punit is an avid thinker and believes in thinking, believing, and acting in line with one’s value to have joy in life. He has developed the philosophy named ‘ABC for joy of life’ which passionately shares. Punit is based out of Belgium, the heart of Europe.
For more information, please click here.
RESOURCES
Listen to the top ranked EU GDPR based privacy podcast...
EK Advisory BV
VAT BE0736566431
Proudly based in EU
Contact
-
Dinant, Belgium
-
hello(at)fit4privacy.com